AI models like ChatGPT are leading the future.
I. Introduction to ChatGPT and Large AI Models
On November 30, 2022, OpenAI released the large language model ChatGPT, which achieved a user base of over a million within 5 days of launch and attracted over 100 million active users within 2 months, setting a record and becoming the fastest-growing application in history.
The explosive popularity of ChatGPT has once again brought artificial intelligence (AI) into the public eye and integrated it with people's work and daily life. The concept of AI can be traced back to the 1950s when pioneers in computer science began to explore the possibility of machines simulating human intelligence. In 1956, at the Dartmouth Conference, this field was officially named "Artificial Intelligence." Early research focused on logical reasoning and problem-solving, but it soon became clear that for machines to be truly intelligent, they must be able to understand and generate natural language.
ChatGPT's "immensely powerful" capabilities are mainly attributed to the large language models it relies on. Initially, research related to natural language processing (NLP) was conducted primarily through the writing of grammatical rules and dictionaries for sentence analysis. In the 1980s, with the improvement of computing power and the emergence of large corpora, statistical methods gradually took the lead, and during this period, many statistical-based machine translation, word segmentation, and part-of-speech tagging methods emerged. With the development of deep learning technology, artificial neural networks and other machine learning methods have made significant progress in the field of natural language processing. From language models based on recurrent neural networks (RNNs) to long short-term memory networks (LSTMs), to large models based on the Transformer architecture, language model performance has continuously improved, gradually achieving capabilities such as text sentiment analysis, natural language inference, dialogue Q&A, contextual learning, and program code generation. In particular, the birth of the Transformer model has rewritten the way language models are constructed, enabling effective processing of long sequences through self-attention mechanisms, greatly enhancing the performance of language models, with subsequent mainstream models all evolving based on the Transformer model.
Advertisement
The emergence of ChatGPT not only marks the arrival of the era of large AI models but also opens a new chapter in the exploration of general artificial intelligence (AGI). Looking back at the development of ChatGPT, the American AI laboratory OpenAI has regarded large language models as the path to AGI since the inception of GPT-1. From GPT-1 to GPT-4, OpenAI's GPT series models have been continuously updated, with each generation's model parameters being an order of magnitude larger than the previous one, with GPT-4's model parameters reaching 1.8 trillion. The model has not only gradually improved in language understanding and reasoning capabilities but has also specifically enhanced its dialogue capabilities for interaction, and the fourth-generation model has added the form of image input. On May 14, 2024, OpenAI released the GPT-4o model, which has further upgraded its performance based on GPT-4. This model is trained end-to-end across text, vision, and audio, with all inputs and outputs processed by the same neural network, increasing response speed and demonstrating the future development trend of large models towards multimodality, further accelerating the pace towards general artificial intelligence.
While the development of large models is in full swing internationally, domestic efforts are also rapidly catching up. The gap between domestic large models and GPT-4 is quickly narrowing, with first-tier leading models such as ChatGLM4, Baidu Wenxin Yiyuan 4.0, and iFlytek Xinghuo V3.5 already performing close to GPT-4, and in the Chinese domain, some domestic models have already reached a level comparable to GPT-4.
ChatGPT's ability to become a new milestone in artificial intelligence is inseparable from the support of computing power. For example, the GPT-3.5 model used by ChatGPT relies on Microsoft's cloud computing for training, with a total computing power consumption of approximately 3640 PF-days (i.e., running at a rate of one thousand trillion calculations per second for 3640 days). This raises a question: as both training and running models require massive computing power, the financial cost has also increased the pressure on company costs. In recent years, as model sizes have continued to expand, training costs have also been increasing. In 2017, the training cost of the Transformer model was about $930. By 2023, the estimated training costs for OpenAI's GPT-4 and Google's Gemini Ultra were about $78 million and $191 million, respectively.
As the current leader in the large model race, OpenAI's business model is mainly divided into three parts: user subscriptions, developer payments, and Microsoft revenue sharing. First, since the beginning of 2023, OpenAI has launched a membership subscription service, charging $20 per month, and by last July, the number of paying users had exceeded 2 million. Second, the developer API interface fee is the core income of OpenAI; under this model, developers use large models to meet their own vertical application scenarios, and OpenAI charges based on traffic. The third part comes from the revenue sharing between OpenAI and Microsoft, selling Azure OpenAI cloud large models to enterprise customers. Successful business model exploration has brought considerable revenue to the company; in 2022, OpenAI still faced a loss of $540 million, but by 2023, the company's revenue had exceeded $1.6 billion.
However, perhaps the main reason for OpenAI's success is building the public's expectations for the promising future of artificial intelligence, with the choice of business model being secondary. In the AI field, there are still countless AI model companies struggling on the brink of survival, and how to explore their own commercialization path is an important challenge they face.
II. How ChatGPT and Other AIs Empower the Financial IndustryThe application of AI in the banking industry is burgeoning, with the robust demand for digital transformation driving both domestic and international banks to invest in areas such as intelligent customer service, smart data assistants, intelligent grading, and intelligent risk control.
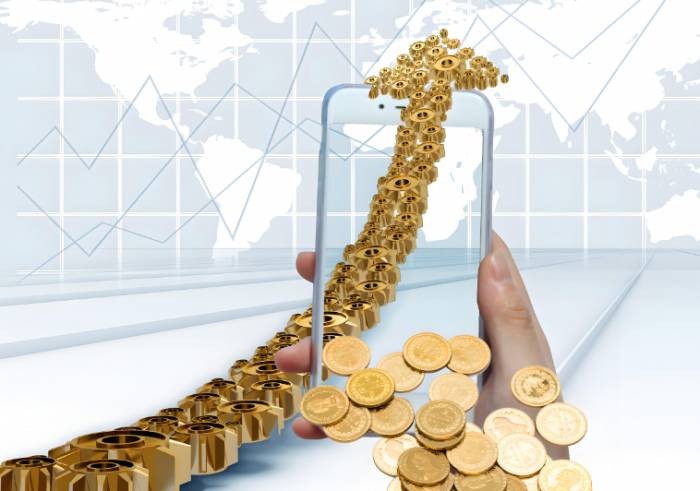
Investment institutions have a more cautious attitude towards ChatGPT. Previously, AI-powered financial models like robo-advisors and AI×quantitative investment have not yet been widely adopted. However, scholars both domestically and internationally have found that ChatGPT is not just a tool but also possesses the capability to guide quantitative trading. In March of this year, research by Sangheum and Cho discovered that ChatGPT could generate buy and sell stock codes based on Twitter news inputs and achieve positive returns. They also found that this result seems to indicate that ChatGPT can process a large amount of non-company-specific news and generate company-specific trading signals accordingly.
In the field of financial technology, following Bloomberg's launch of the financial vertical large model BloombergGPT in March 2023, a wave of financial vertical large models has emerged both domestically and internationally. Compared to general-purpose large models, financial vertical large models are better suited to the financial industry's strict regulatory requirements, high precision demands, and risk-averse characteristics.
III. The Future of AI×Finance and Recommendations
From a macro perspective, AI×finance is key to the strategic layout of financial institutions in the coming years. AI technology, regarded as the "important engine of new quality productivity," will serve as the foundation and capability of digital finance and deeply integrate with the central emphasis on the "five major articles of finance."
In the field of financial regulation, AI is both a regulatory tool and a regulatory target. Currently, there are two main issues with the application of AI in finance. On the technical level, first, as a service industry with strict compliance requirements, how can finance reduce the risk of AI outputting "toxic content"? Second, how can the financial industry, which has strict confidentiality requirements, protect data privacy in the AI era? Therefore, the new AI×finance model also requires new monitoring methods to match. AI can quickly obtain a large amount of diversified data from multiple channels, which helps to form a comprehensive regulatory force and achieve "synchronization" of development and regulation.
Looking at the sub-fields of the financial industry, a host of AI large models, led by ChatGPT, will gradually integrate more deeply with various business areas. In the research field, the latest version of ChatGPT, GPT-4o, has introduced online data document analysis capabilities, allowing direct upload of Excel files for analysis. Analysts no longer need to manually input Excel functions or write code themselves; they can obtain a large number of indicators through GPT-4o's online analysis. In the field of quantitative investment, ChatGPT will not only serve as a tool to liberate productivity, helping quantitative practitioners reduce the time and effort spent on basic work, but also optimize the logic of quantitative investment through large model coupling and large model emergence. In the future, the analytical assistance functions that ChatGPT and various AI large models can undertake may become even more powerful. For example, Li Wen et al. (2024) proposed that ChatGPT+RPA could achieve risk control of margin trading collateral assets, indicating that there is still much room for expansion and deepening of ChatGPT's application in the financial field.
Leave A Comment